Theory of Machine Learning
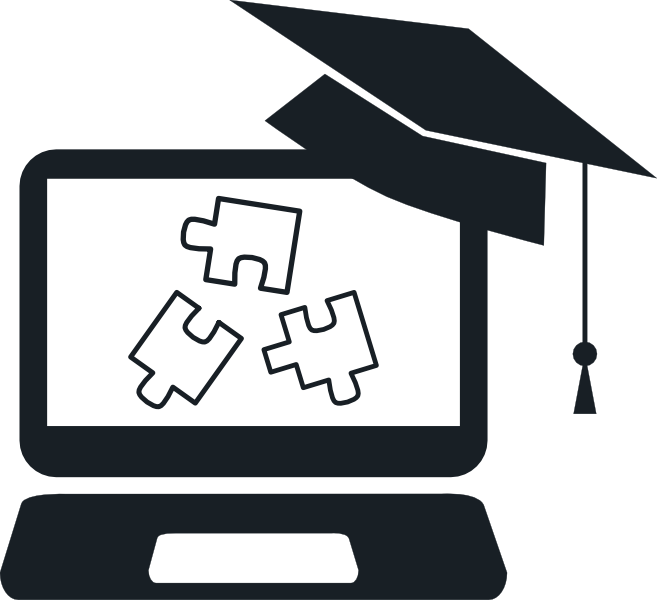
Beside practical applications, the Group has also had a long history of theoretical research. Current research projects are carried out in the field of theory revision. In this scenario, an initial hypothesis is available at the onset of learning (e.g. a system constructed by experts), which is far from being a perfect model of the system, but it is a good approximation. Further research is being carried out in order to relate the purely logical approach (belief revision) to application-oriented approach (machine learning). The group also investigated the properties of large networks. The betweenness centrality measures the centrality of a node in terms of the paths traversing it. Recently its k-bounded variant was introduced, which is only concerned with the paths of length at most k. The betweenness profile of a node consists of its k-bounded betweenness centrality values. The group investigated various properties of this profile in the case of trees under the worst-case scenario, and in the case of scale-free random trees. Lastly, the team made progress on the topic of optimizing the AUC (Area Under the ROC Curve) online. The group introduced a new class of directed hypergraphs and provided worst case estimations for the centrality profile in trees. A new approach is provided for the evaluation of knowledge bases based on the ConceptNet.
People
György Turán (contact), Gábor Berend