Speech Technology
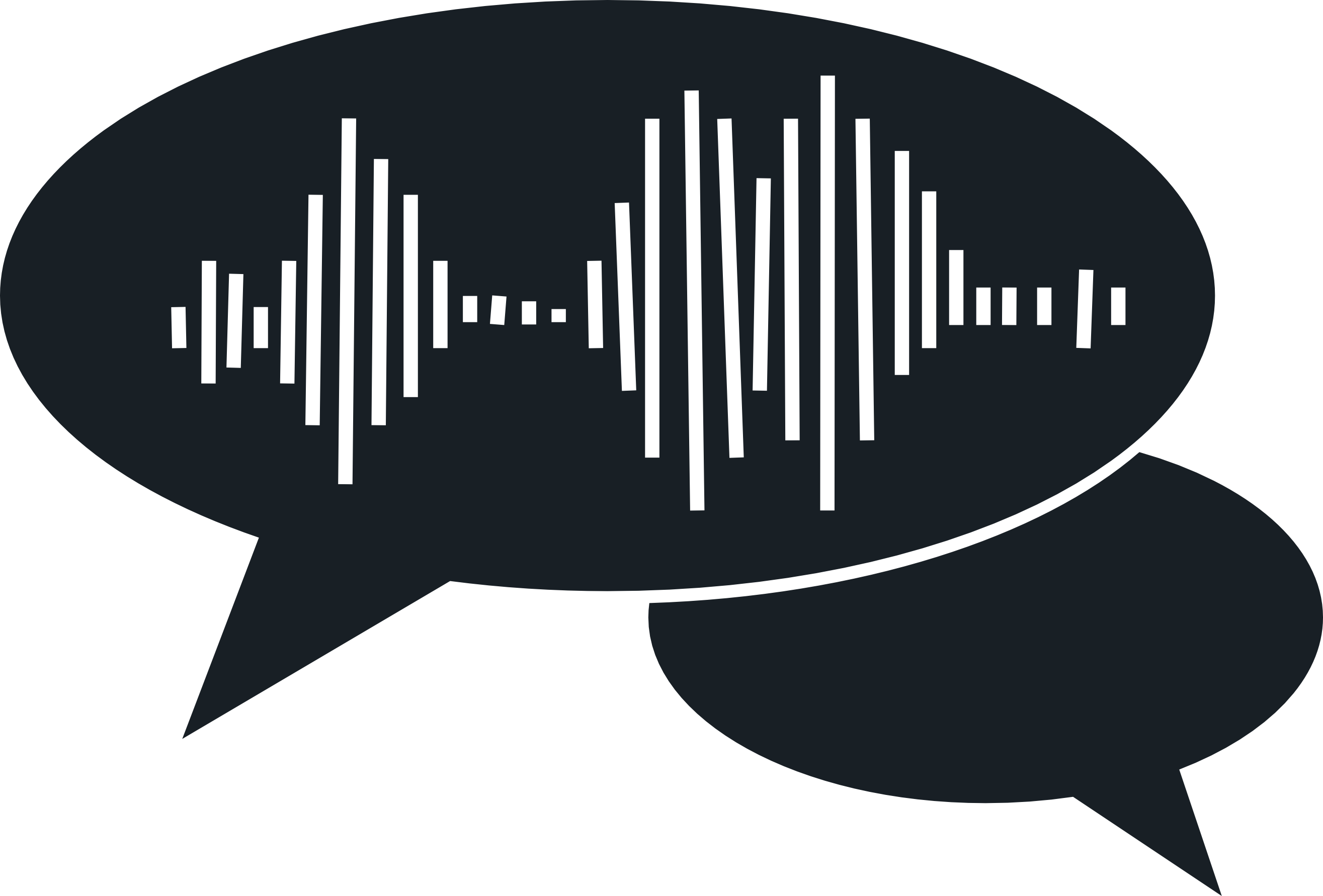
From among the numerous topics of speech technology, our team focuses on those that require the use of machine learning. As a result, our main topic of interest is speech recognition, which has been known to apply statistical pattern recognition methods since the 80s.
We started developing neural network-based solutions in the 90s, therefore, at the advent of deep learning, we were quickly able to join this new direction. With our own DNN implementation package we are able to produce recognition results that compete with international publications. We seek to incorporate the newest technological advancements into our code (e.g. new activation functions, convolutional neural nets, batch normalization, recurrent networks and so on) as quickly as possible. Due to the fact that we need to produce international publications, we usually train our systems on English datasets. At the same time, we are also involved in continuously increasing the accuracy of our Hungarian recognition system. With this latter aim in mind, we developed a recognition system for Hungarian broadcast news, along with a decent-sized database of broadcast news recordings. Besides speech recognition, we are interested in all aspects of speech technology that can be handled by machine learning.
Recently, we have been focusing on medical applications. For example, we achieved compelling results in the detection of early Alzheimer's disease from speech signals, and in the area of silent speech interfaces, the latter of which deals with the reconstruction of the speech signal from ultrasound movies of the tongue movement.
Prizes Interspeech ComParE Challenge 2013, Emotion Sub-Challenge: First prize. Interspeech ComParE Challenge 2015, Parkinson's Sub-Challenge: First prize. Interspeech ComParE Challenge 2017, Cold Sub-Challenge: First prize. Interspeech ComParE Challenge 2018, Crying Sub-Challenge: First prize.
People
Gábor Gosztolya (contact), László Tóth, György Kovács, Ádám Pintér, Mercédesz Vetráb
Projects
Silent speech interfaces This area focuses on recognition and synthesis of silent speech, which is a form of spoken communication where the subject is just silently articulating without producing any sound. Our team uses ultrasound tongue imaging (UTI) as the input; our aim is to re-synthesize the speech from the ultrasound video only via the use of Deep Learning. Partners: Budapest University of Technology and Economics; Eötvös Loránd University, Budapest
Medical applications It is well-known that many neurogical and physical disorders change the speech of the subject (e.g. Alzheimer's Disease or Parkinson's Disease). Some such changes can be detected at a quite early stage, and a timely diagnosis might allow to slow the progress of the disease. Our group focuses on such automatic detection of various diseases (e.g. Alzheimer's Disease, Mild Cognitive Impairment, Parkinson's Disease, Schizophrenia) from the subject's speech via machine learning tools, i.e. by utilizing different machine learning methods and developing new feature sets. Partners: Department of Psychiatry, University of Szeged; Research Institute for Linguistics, Hungarian Academy of Sciences, Budapest
Paralinguistic Research In the past decade, within speech technology more attention is paid to the analysis of the non-verbal content of human speech. Of course, some tasks (such as emotion identification or laughter detection) have a longer history, but recently several other tasks have gained attention (e.g. estimating the level of conflicts, determining the gender, age or weight of the speaker). Our team focuses on the application of deep learning in these tasks, both in the feature extraction and in the classification steps.